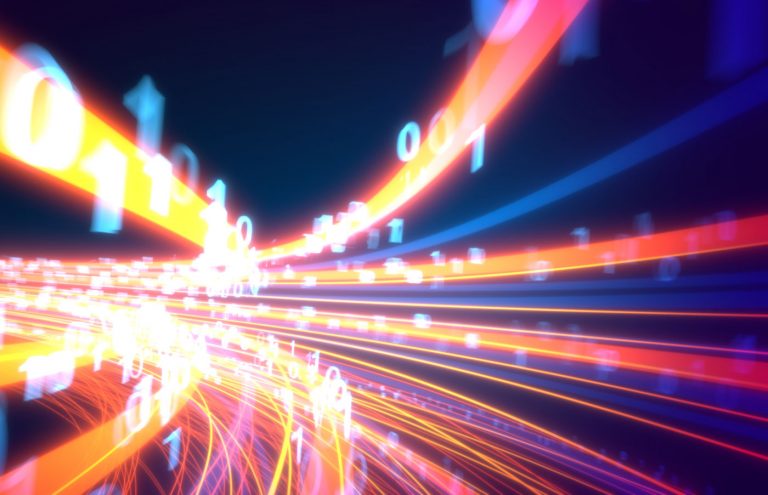
A prototype AI tool developed by scientists in the U.S. and China has demonstrated, in small-scale study, that it can accurately predict which newly infected patients with SARS-CoV-2 would progress to develop severe respiratory problems as a result of the disease.
Surprisingly, the study found that characteristics of COVID-19, including fever, certain features in radiographic lung images, and strong immune responses, weren’t useful in predicting which of the 53 patients—all presenting with initially mild symptoms—would progress to develop severe lung disease. Neither was age or gender helpful in predicting the development of serious disease. Instead, the results indicated that a combination of small changes in levels of the liver enzyme alanine aminotransferase (ALT), patient-reported myalgia (aching muscles), and raised hemoglobin levels, most accurately predicted the development severe disease. Together with other factors, the team reported being able to predict risk of acute respiratory distress syndrome (ARDS) with up to 80% accuracy.
“Our goal was to design and deploy a decision-support tool using AI capabilities—mostly predictive analytics—to flag future clinical coronavirus severity,” said Anasse Bari, PhD, a clinical assistant professor in computer science at the Courant institute, and co-author of the researchers’ paper in Computers, Materials & Continua. “We hope that the tool, when fully developed, will be useful to physicians as they assess which moderately ill patients really need beds, and who can safely go home, with hospital resources stretched thin.”
Bari, Megan Coffee, MD, PhD, clinical assistant professor, department of medicine at NYU, and colleagues report on their development in a paper titled, “Towards an Artificial Intelligence Framework for Data-Driven Prediction of Coronavirus Clinical Severity.” Development of the AI tool was led by researchers at NYU Grossman School of Medicine, and the Courant Institute of Mathematical Sciences at New York University, in partnership with Wenzhou Central Hospital and Cangnan People’s Hospital.
As of March 30, the SARS-CoV-2 virus had infected 735,560 patients worldwide, Bari et al., wrote. According to the World Health Organization, the illness has caused more than 34,830 deaths, more often among older patients with underlying health conditions. Symptoms commonly will begin as mild in all patients, and include cough, fever, and occasional dyspnea, without any sudden onset of severe disease, but in a minority of patients severe symptoms do develop at about day 5–8, including shortness of breath, and pneumonitis, potentially progressing to ARDS. And while 80% of COVID-19 cases are mild, more serious caseloads can overwhelm hospitals, the authors continued, with high requirements for oxygen, prolonged ventilation, and sometimes extracorporeal membrane oxygenation (ECMO), particularly for patients with ARDS.
While we know that severe COVID-19 infections are relatively more likely to be seen in men, and the risk increases with age over 50 years, it can be difficult to predict who is at greatest risk of developing severe disease. “Faced with the initial mild presentation of COVID-19 in patients, it may be difficult to identify who will more likely develop severe illness by using established risk factors alone such as age, gender, and comorbidities,” the authors wrote. The ability to predict which patients are more likely to develop ARDS, and so are at greatest risk of complications and potentially death, would help triage. “This is particularly important in a novel and accelerating outbreak when critical care resources and hospital beds are limited, and clinicians are forced to make difficult decisions without past specific experience to guide clinical acumen.”
The investigators explored whether the use of data available on patients at initial presentation could be used to develop an AI tool that could provide clinical decision support. “… AI has begun to tackle these difficult challenges in healthcare, and can provide clinical decision support if used carefully,” the team pointed out. For example, AI tools can predict the risk of heart attack from retinal images, risk stratify Ebola patients, and screen chest images for tuberculosis, or mammograms for cancer. Decision trees, a predictive analytics technique used in this study, have been previously used for pneumonia risk prediction.”
The goal of the researchers’ newly reported study was to determine whether AI techniques could help to accurately predict which patients with SARS-CoV-2 would go on to develop potentially fatal ARDS. The team collected laboratory and radiological findings from 53 patients who tested positive for SARS-CoV-2, during January 2020, at the two Chinese hospitals. Infection was detected in throat swab samples using a real-time reverse transcription polymerase chain reaction (RT-PCR) assay. The median age of the patients was 43 years, and there were 33 men and 20 women. Symptoms were typically mild to begin with, and included cough, fever, and stomach upset, although other symptoms reported in some patients included myalgia, wheezing, dyspnea, nasal congestion, and sore throat. In a minority of patients, severe symptoms, including pneumonia, developed within a week.
The researchers designed computer models that make decisions based on the data fed into them, with programs getting smarter the more data they considered. Specifically, the study used decision trees that track series of decisions between options, and that model the potential consequences of choices at each step in a pathway.
“Predictive analytics (a form of artificial intelligence) learns from historical data to help predict future outcomes,” the investigators explained. “The technology uses machine learning algorithms that can extract insights and rules from experience (historical examples) in order to determine data attributes (features) with the most predictive power for making accurate predictions.” In predictive analytics, they noted, a feature (also known as variable, or observation), is an individual measurable attribute. For their studies, the investigators included the available features for each patient, such as physical symptoms, radiological findings, and comprehensive blood analysis data, such as levels of immune system cells and hemoglobin.
The resulting AI model found, surprisingly, that features considered to be hallmarks of COVID-19 in fact weren’t the most useful in predicting which patients would progress to ARDS. “Characteristics that are hallmarks of COVID-19 diagnosis, such as ground glass opacities on chest computed tomography, as well as fever, cough, and lymphopenia, assist in initial clinical diagnosis,” the team commented. “However, given uniformity, these did not distinguish risk of disease progression and were not highly predictive … Other factors like dyspnea on presentation, which would have logically been expected to correlate with ARDS, did not feature as predictive in this model, as this was common in many patients.”
Instead, the new AI tool found that the most accurate predictor of severe disease was a combination of changes in three features—levels of ALT, reported myalgia, and higher hemoglobin levels. “The features that machine learning showed best predicted ARDS were not the indicators a clinician would standardly select, nor were these values grossly abnormal clinically,” the investigators acknowledged. “Multiple iterations showed that the most predictive features included an increase in alanine aminotransferase (ALT) and hemoglobin, and the presence of myalgias.”
In fact ALT levels—which rise dramatically as diseases like hepatitis damage the liver—were only a bit higher in patients with COVID-19, the researchers said, but still featured prominently in the prediction of severity. Deep muscle aches were also more commonplace, and have been linked by past research to higher general inflammation in the body. And while smoking has long been linked with raised hemoglobin levels, of the 33 patients at Wenzhou Central Hospital interviewed on smoking status, the two who reported having smoked, also reported that they had quit.
Limitations of the study, the authors acknowledged, included the relatively small data set and the limited clinical severity of disease in the population studied. The latter may be due in part to an as yet unexplained dearth of elderly patients admitted into the hospitals during the study period. The average patient age in the reported was 43 years, and none of the patients was over 67 years of age. For this patient cohort, the models were nevertheless 70–80% predictive. The team noted that additional studies will be needed to help improve the model. “Further validation and refinement of this model for a wider clinical spectrum will require further clinical datasets with diverse disease presentations, which we look to include in the near future.”
The investigators suggested that their study demonstrates that predictive analytics could feasibly be used alongside doctors to help distinguish “sick” from “not sick,” and that the model highlights how some pieces of clinical data may be “underappreciated” by clinicians, such as small increases in ALT and hemoglobin as well as myalgia. However, they stressed, “Just as predictive text is intended to augment, but not replace writers, the goal is not to create a black box to supersede clinical reasoning, but to create models that can provide insight … Further refinement of these models with more data, from different settings with different spectrums of severity, would strengthen the predictive power of the model and allow it to be a useful tool.”
“While work remains to further validate our model, it holds promise as another tool to predict the patients most vulnerable to the virus, but only in support of physicians’ hard-won clinical experience in treating viral infections,” said Coffee. “I will be paying more attention in my clinical practice to our data points, watching patients closer if they, for instance, complain of severe myalgia. It’s exciting to be able to share data with the field in real time when it can be useful. In all past epidemics, journal papers only published well after the infections had waned.”