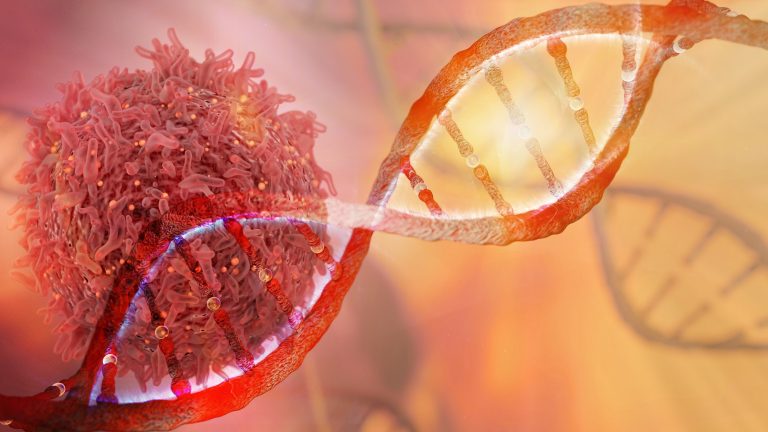
Making meaningful connections between high-throughput molecular data and health implications poses significant challenges, many of them computational. But now a team based at the Max Planck Institute for Molecular Genetics (MPIMG) in Berlin and at the Institute of Computational Biology of Helmholtz Zentrum München has developed a new algorithm using machine learning technology for that purpose. Known as Explainable Multi-Omics Graph Integration (EMOGI), the machine learning method predicted 165 new cancer genes by combining multiomics pan-cancer data—such as mutations, copy number changes, DNA methylation and gene expression—together with protein–protein interaction (PPI) networks.
“Genetic as well as non-genetic causes contribute to tumorigenesis, and this necessitates the development of predictive models to effectively integrate different data modalities while being interpretable,” the authors write in a paper in Nature Machine Intelligence.
“In this case, it is a nice example of interpretable AI to gain mechanistic insights into predictions and enable (hopefully!) in the future the use of machine learning models in the clinic,” said co-author Annalisa Marsico, former head of a research group at the MPIMG
In this study, the EMOGI the software analyzed tens of thousands of different network maps from 16 different cancer types from data sets patient samples. These 12,000-19,000 data points contain information about DNA methylations, the activity of individual genes and the interactions of proteins within cellular pathways in addition to sequence data with mutations. In these data, a deep-learning algorithm detects the patterns and molecular principles that lead to the development of cancer.
“This is the foundation for personalized cancer therapy,” said Marsico.
Molecular data on pathogenic gene sequence changes have long been the focus for identifying cancer-causing genes. But increasingly, research shows epigenetic changes or otherwise dysregulated gene activity contribute to cancer as well.
For this reason, the researchers sought a new tool to merge sequence data – the main drivers of cancer – with other cellular information. “We used layer-wise relevance propagation to stratify genes according to whether their classification was driven by the interactome or any of the omics levels, and to identify important modules in the PPI network,” they write. With EMOGI, they could confirm the presence of driver mutations but also pinpoint other gene candidates that are in less direct contact to the actual cancer-driving gene.
The proposed 165 new cancer genes discovered via EMOGI did not necessarily harbor recurrent alterations but did interact with known cancer genes. Further study showed they were linked with essential genes from loss-of-function screens.
As an example, the team found genes whose sequence is mostly unchanged in cancer, and yet are indispensable to the tumor because they regulate energy supply. These genes are dysregulated by other means, such as chemical changes on the DNA like methylation, that influence a gene’s activity.
“Such genes are promising drug targets, but because they operate in the background, we can only find them by using complex algorithms,” they write.
The EMOGI program is not limited to cancer, the researchers emphasize. Says Marsico, “It could be useful to apply our algorithm for similarly complex diseases for which multifaceted data are collected and where genes play an important role. An example might be complex metabolic diseases such as diabetes.”