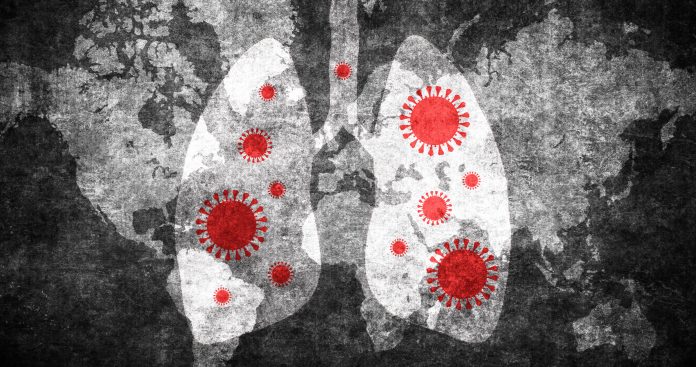
Research shows that combining computed tomography (CT) scanning with artificial intelligence (AI) can help to visualize hard to image lung damage caused by respiratory conditions such as COVID-19.
The new method, developed by scientists at King Abdullah University of Science and Technology (KAUST) in Saudi Arabia and NorthEast Forestry University, Harbin Medical University and Mudanjiang Medical University in China, should help clinicians treat patients with such respiratory conditions and also to better predict their long-term prognosis.
Conventional CT scans struggle to pick up some lung abnormalities that result from COVID-19 or other respiratory illnesses. “Survivors who had severe symptoms… have much worse six-months follow-up lung function than the mild-symptom patients, whereas their six-month follow-up CT scans are very similar from almost all aspects,” write Xin Gao, a professor at KAUST, and colleagues in the journal Nature Machine Intelligence.
To try and help improve imaging for COVID-19 patients and those with other lung conditions, Gao and team created a type of imaging they call Deep-Lung Parenchyma-Enhancing (DLPE). They used AI algorithms to find and enhance hard to visualize lung damage from CT scan data.
“COVID-19 often causes pulmonary parenchyma lesions months after discharge, such as ground glass opacities, consolidations and long-term fibrosis,” explain the researchers. By removing non-informative tissues from the scan, DLPE was able to clearly pick up such lung problems.
The researchers first trained and validated their algorithms using data from 3644 CT scans of COVID-19 patients in China. They then tested the technology in a group of over 1000 inpatients with COVID-19 and used it to predict long term outcomes in 219 survivors, 69 of whom initially had severe disease.
“On the inpatient CT dataset, DLPE found novel subvisual lesions that resemble fainter ground-glass opacities, which may reflect mild plasma fluid leakages due to disruption of the epithelium of alveolar,” explain the authors.
In the survivor cohort, many sub-visual abnormalities were found using DLPE, most likely reflecting mild pulmonary fibrosis. Before the AI algorithm was applied around 3.5 cm3 of lesions were found in each survivor on average, after DLPE this increased to 109cm3.
“These subvisual lesions have strong correlations with sequelae related to fibrosis: more subvisual lesions means lower lung capacity, less alveolar-capillary gas conductance and a worse St George’s Respiratory Questionnaire score, which are all typical consequences of pulmonary fibrosis,” write the authors, who say the new technique could help explain why many COVID-19 survivors still have respiratory symptoms, despite apparently normal looking lung scans.
Although the first use of DLPE was in COVID-19 patients, the research team also tested the technology in some patients with pneumonia, tuberculosis and lung cancer.
“DLPE can make robust enhancement and critical segmentation for various lung diseases, which demonstrates its generalization power and potential clinical usefulness,” they conclude.