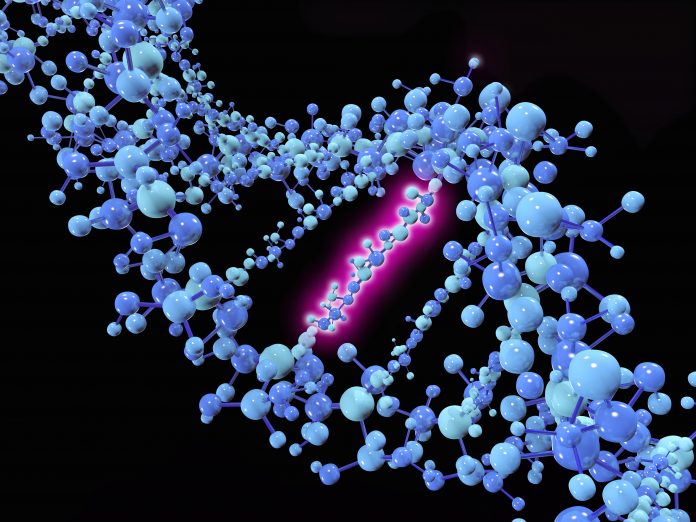
Researchers at Children’s Hospital of Philadelphia (CHOP) have developed a new tool to help researchers interpret the clinical significance of somatic mutations in cancer. The tool, known as CancerVar (Cancer Variants interpretation), incorporates machine learning frameworks to interpret the potential significance of those mutations in terms of cancer diagnosis, prognosis, and targetability. A paper describing CancerVar was published in Science Advances.
Several knowledge bases, such as CIViC and OncoKB, have been manually curated to support clinical interpretations of a limited number of “hotspot” somatic mutations in cancer. “However most of these knowledge bases were built for well-studied mutations for which knowledge is already extensive,” said senior author Kai Wang, Ph.D., Professor of Pathology and Laboratory Medicine at CHOP. “The idea of building CancerVar is more for addressing somatic mutations that are previously unreported in a hotspot site.” The mutation could be an oncogene, a tumor suppressor, or something differently. “The goal of CancerVar is to supplement the existing knowledge bases and use machine learning approaches to predict the possible oncogenicity of these new variants or previously unreported variants,” he adds.
Wang and colleagues focused on slightly over 1,900 genes that are implicated in cancer and then mutated every single permutation of this gene and grouped them into one of four tiers. With this method the team pre-computed over 12.9 million somatic mutations ranking them as having strong clinical significance, potential clinical significance, uncertain clinical significance, and benign/likely benign, based on the AMP/ASCO/CAP 2017 guideline—a jointly proposed standards and guidelines for interpreting, reporting, and scoring somatic variants.
“Now, in the future, if somebody finds a new mutation that’s causing a single base change, they can search the CancerVar database to see it’s precomputed score and predict the mutation’s likely significance,” said Wang.
CancerVar uses the Python command-line software with an accompanying web server to provide clinical evidence for the nearly 13 million somatic cancer variants. The CancerVar web server provides multiple query options at variant, gene, and copy number alteration (CNA) levels across 30 cancer types and two versions of reference genomes: hg19 (GRCh37) and hg38 (GRCh38).
CancerVar relies on a deep learning-based scoring system to predict oncogenicity of mutations by the semi-supervised generative adversarial network (SGAN) method using both functional and clinical evidence. In this study, Wang describes training and validating the SGAN model on 5,234 somatic mutations from an in-house database of clinical reports from the cancer diagnostic lab at CHOP. They also found good concordance when evaluating 6,226 variants that were curated through a literature search.
“This new tool can facilitate human reviewers in drafting clinical reports and provide guidance on whether the mutations are oncogenic or not and otherwise help researchers and clinicians prioritize mutations of concern,” says Wang. Instead of independently sorting through variants identified through sequencing and drafting clinical report, users can input the mutation of interest into the CancerVar database and make their own judgement on its clinical significance based on professional guidelines. The results can summarized and inserted into a paragraph to be used in a paper or in a report.
Additionally, that web server provides functionality to finetune the evidence, given some of the current guidelines are somewhat ad hoc. “Users may want to tweak it to put more weight or emphasis on a particular aspect depending on the type of cancer they are trying to interpret,” adds Wang. “CancerVar gives this flexibility to get to the final predictions.”