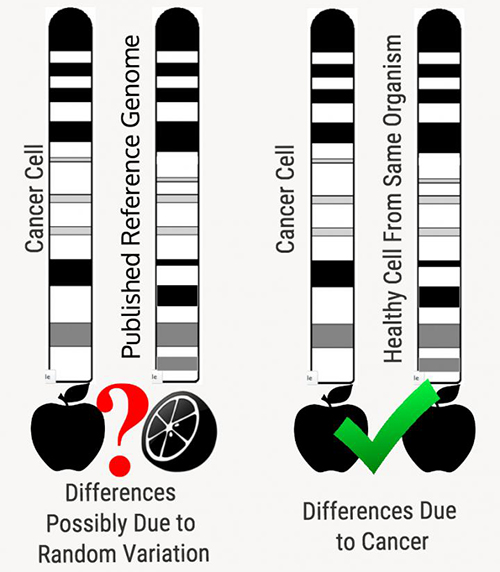
Investigators at the University of Colorado (CU) Cancer Center came across unexpected findings recently, while trying to detect genomic rearrangements in cancer genomes while utilizing their mouse model of B cell lymphoma. To their surprise, the Colorado researchers found more than 1,000 genetic translocations in their mouse model, a result that they initial dismissed as experimental error. The findings from this new study were published recently in BMC Genomics through an article entitled “Unexpected effects of different genetic backgrounds on identification of genomic rearrangements via whole-genome next generation sequencing.”
To rule out experimental technique as the cause of the way-more-than-expected genomic alterations, the CU researchers sequenced three different types of cells from wildtype mice. However, like the lymphoma cells before them, the cells from wildtype mice also had over a 1,000 translocations. Still unconvinced that they weren’t witnessing experiential phenomena, the scientists decided to try one last ditch effort.
“We thought 'let's just do another practice,'” remarked senior study investigator Jing Hong Wang, M.D., Ph.D., associate professor in the department of immunology and microbiology at CU School of Medicine.
For “practice,” the CU team downloaded new mouse genomic data from the Wellcome Trust Sanger Institute, one of the world's leading institutes for genetic research.
“When we mapped the genome of this particular mouse strain against the mouse reference genome published by the National Center for Biotechnology Information, we found thousands of translocations, even more than our experimental model!” Dr. Wang exclaimed.
The CU team began to realize that the issues did not lie with their experimental mouse, the quality of their data, or the computational algorithm they used to discover translocations. The problem, as they outlined in the paper, was that reference genomes are different for various mouse strains. Not all mice have the same DNA sequences in the same locations on their chromosomes. Because of this genetic variation, the DNA sequences of one mouse strain may appear out of place when compared with the DNA sequences of any other mouse strain.
“Unfortunately, when we have so many events, the artifacts may mask our real events,” Dr. Wang noted, meaning that with thousands of translocations identified by next-generation sequencing, it was almost impossible to discover the “needle” of a potentially oncogenic translocation amid the “haystack” of identified translocations that were, in fact, only the unimportant, random differences between individual mouse genomes.
“Then we started to think about all these human cancer genomic studies,” Dr. Wang added. “People use all this sequencing data to show genomic changes in human cancers, but what if these studies have similar comparison problems?”
For next generation sequencing, machines read a test genome as an array of small DNA fragments, each made up of 100 to 150 base pairs—which are then aligned to the reference genome like puzzle pieces. When there is a match, the system puts the piece in place and thus and can fill in the rest of the sequence from the knowledge already gained by the reference genome.
Unfortunately, with 3 billion base pairs in the human genome, there may be many false matches for short, 100 base-pair snips. Yet many researchers are optimistic that new long read (1,000 or more base pairs) sequencing technology is on the way to solve this problem.
Until then, Dr. Wang proposes a possible fix: “We suggest considering not mapping your data to a reference genome, but to the genome of some cell from the same source that doesn't have cancer,”, i.e., de novo assembly. By way of analogy, instead of comparing a cancerous apple to a healthy orange, it is comparing a cancerous apple to a healthy apple (see figure above).
“People should be their own control. Instead of working with the published, generic reference genome, we should work with two samples (control vs. cancer) from the same person,” Dr. Wang concluded. “Only then can you really figure out what's going on in your cancer cell genome.”