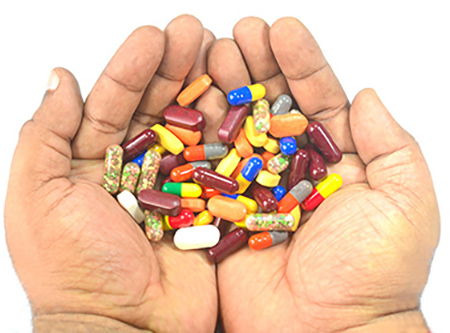
Electroencephalography (EEG) could help predict whether someone will benefit from a specific antidepressant medication, research suggests.
A predictive model based on electrical activity readings in the brain was able to determine response to two distinct selective serotonin reuptake inhibitors (SSRIs) in more than 60% of the cases studied.
The findings could help match patients with the most effective treatment and potentially help personalize it, the researchers suggested in JAMA Network Open.
“Accurately predicting treatment response in almost two-thirds of cases could lead to improved patient outcomes, reducing ineffective treatments and health care burden,” pointed out Faranak Farzan, PhD, from Simon Fraser University in Canada, and colleagues.
Major depressive disorder (MDD) is a leading cause of disability, affecting more than 300 million people worldwide.
Although antidepressant medications are prescribed as a first-line treatment, remission after trial periods for first and second medications can occur in 35% to 50% of patients, with further reductions in subsequent trials.
Each trial can take up to 12 weeks and predicting medication response could shorten the duration of illness and its associated negative outcomes.
Yet existing EEG-based models for predicting antidepressant response have lacked adequate external validation or relied on high-density EEG devices that require extensive training and expertise.
In order to develop a model with better clinical viability, Farzan and co-workers used EEG data collected between 2011 and 2017 from two independent cohorts of individuals with depression.
Firstly, they adapted an EEG-based model for predicting antidepressant response using data on 125 individuals in the Canadian Biomarker Integration Network in Depression (CAN-BIND) study to make it more suitable for a clinical environment.
Participants in that study received open-label escitalopram treatment across six clinical sites, with resting-state, eyes-closed EEG data collected using high-density EEG devices.
An earlier study had used features derived from 58 common electrodes across sites at baseline and week two to develop a previous model.
But for the current study, with feasibility in mind, Farzan and team used clinically relevant baseline data with a selection of 32 electrodes grouped in 14 brain regions to emulate a setup closer to a clinical environment.
They then obtained external validation for their findings using an independent data set from 223 participants in the Establishing Moderators and Biosignatures of Antidepressant Response for Clinical Care (EMBARC) for Depression consortium.
In that study, 105 individuals were randomly assigned to sertraline and 118 to placebo across four clinical sites.
Using internal validation with CAN-BIND, the model achieved 64.2% balanced accuracy, and this was confirmed with the 63.7% accuracy found using external validation with EMBARC.
“This finding is of clinical importance because it provides a substantial improvement over trial-and error methods,” the authors noted.
They concluded: “These findings represent a substantial advancement toward using EEG in future clinical practice and supporting its potential to match patients with MDD to optimized treatment.”