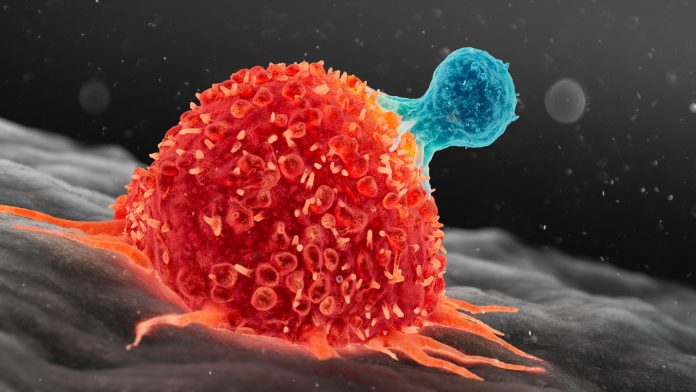
A gene signature can accurately predict the functioning of p53 variants, according to work by researchers at The Wistar Institute and their colleagues. Long a focus of drug discovery efforts, the p53 tumor suppressor protein normally protects against cancer initiation and progression. Disrupted p53 is linked to cancer, but there are so many variations in this gene it has been difficult to interpret each of their clinical significance.
The team’s findings could be used to screen individuals for genetic variants of p53 that help predict cancer risk and possible response to therapy. Their study appeared this week in PNAS.
“The promise of this research is personalized medicine,” said Maureen E. Murphy, PhD, senior author and Program Leader in Wistar’s Molecular & Cellular Oncogenesis Program.
Cancer is a leading cause of death worldwide. Because early detection is often critical to surviving this disease, there is tremendous need for tests that can find cancers as soon as possible. Dozens of companies are working on this and the worldwide market for cancer diagnostics is currently estimated to be worth more than $150 Billion and expected to grow more than six percent per year over the next few years. Over 20,000 trials of cancer tests are listed on clinicaltrials.com.
Biochemically, p53 functions primarily as a sequence-specific transcription factor. Mutations in the TP53 gene disrupt the tumor suppression by its encoded protein, p53. These are the most common single gene alterations in human cancers—hundreds of missense single nucleotide polymorphisms (SNPs) may alter p53 function. Some of these variants are recognized as drivers of certain tumors because they undo the tumor suppressor function.
“There are so many genetic variants of P53,” explained Murphy. “A lot of P53 variants are classified as having uncertain significance with current methods of testing. This does not help people determine whether they have increased cancer risk. The signature we identified does.”
This team monitored differences in activity in mutant and normal p53 proteins to determine any genetic markers that would flag if a p53 variant is less functional than normal. In collaboration with Andrew Kossenkov, PhD, assistant professor in Wistar’s Vaccine and Immunotherapy Center, the team used machine learning to identify a gene signature that consistently and accurately predicted the difference between a normal functioning or benign p53 and a lesser functioning variant of the protein.
Murphy said she intends to continue this work with the goal of turning the gene signature into a blood-based genetic test that could help people learn about their p53 status.