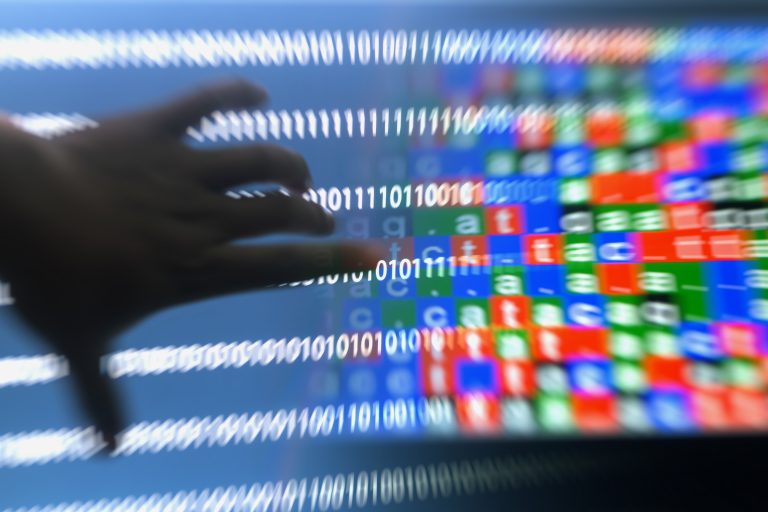
A Nature Methods paper out this week details a new approach to gene expression data analysis that could greatly increase the information available from such studies. The team, from AI pioneer DeepMind and Alphabet company Calico, has developed a new model based on Transformers, which are common in natural language processing. The tool, Enformer, is a neural network architecture. According to the study, it led to greatly increased accuracy in predicting gene expression from DNA sequence.
“We report substantially improved gene expression prediction accuracy from DNA sequences through the use of a deep learning architecture, called Enformer, that is able to integrate information from long-range interactions (up to 100 kb away) in the genome,” the authors write.
The paper, “Effective gene expression prediction from sequence by integrating long-range interactions,” was first shared as a preprint on bioRxiv. The lead author is Žiga Avsec, senior research scientist at DeepMind. “To advance further study of gene regulation and causal factors in diseases,” the team also made their model and its initial predictions of common genetic variants openly available here.
Earlier gene expression analytical approaches have mainly been based on convolutional neural networks, but these have limitations in modelling the influence of distal enhancers on gene expression, and that has hindered their accuracy and application.
These deep convolutional neural networks (CNNs) are the “state of the art” at predicting gene expression from DNA sequences for the human and mouse genomes. But these models can only take into account sequence elements up to 20 kb away from the transcription start site because the locality of convolutions limits information flow in the network between elements farther apart.
However, well-studied regulatory elements, including enhancers, repressors, and insulators, can influence gene expression from far greater than 20 kb away. Thus, it makes sense that adding information from distal elements should help increase predictive accuracy.
The team’s initial explorations relied on Calico’s Basenji2, which predicted regulatory activity from relatively long DNA sequences of 40,000 base pairs.
But the team wanted to go further than that. On the Deep Mind blog, Avsec writes, “Motivated by this work and the knowledge that regulatory DNA elements can influence expression at greater distances, we saw the need for a fundamental architectural change to capture long sequences.”
The aim, Avsec adds, is to “make use of self-attention mechanisms that could integrate much greater DNA context.”
He also writes that: “Transformers are ideal for looking at long passages of text; we adapted them to “read” vastly extended DNA sequences. By effectively processing sequences to consider interactions at distances that are more than 5 times (i.e. 200,000 base pairs) the length of previous methods, our architecture can model the influence of important regulatory elements called enhancers on gene expression from further away within the DNA sequence.”
Their research showed this approach yielded more accurate variant effect predictions on gene expression for both natural genetic variants and saturation mutagenesis measured by massively parallel reporter assays. Furthermore, Enformer learned to predict enhancer–promoter interactions directly from the DNA sequence competitively with methods that take direct experimental data as input.
“We expect that these advances will enable more effective fine-mapping of human disease associations and provide a framework to interpret cis-regulatory evolution,” the researchers wrote.