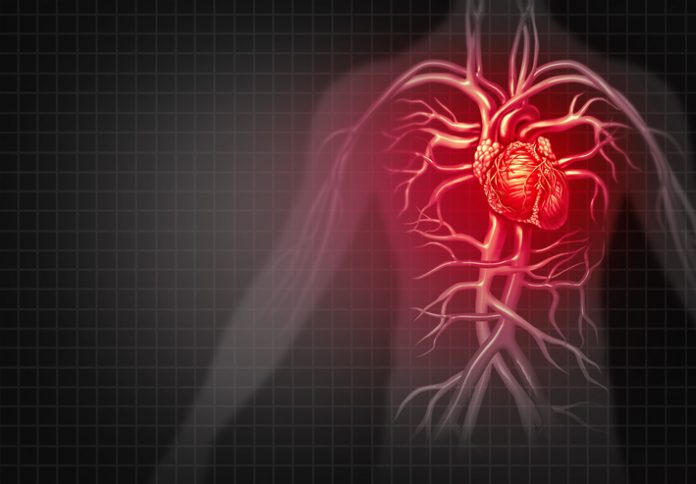
Researchers have developed a deep learning tool that predicts a patient’s 10-year risk of death from a heart attack or stroke using a single X-ray. Results of the study were presented this week at the annual meeting of the Radiological Society of North America (RSNA).
The team’s CXR-CVD risk model performed similarly to the established clinical standard and even provided incremental value.
“Our deep learning model offers a potential solution for population-based opportunistic screening of cardiovascular disease risk using existing chest X-ray images,” said the study’s lead author, Jakob Weiss, MD, a radiologist affiliated with the Cardiovascular Imaging Research Center at Massachusetts General Hospital and the AI in Medicine program at the Brigham and Women’s Hospital in Boston. “This type of screening could be used to identify individuals who would benefit from statin medication but are currently untreated.”
The American Heart Association reports that just over 19 million people died worldwide from cardiovascular disease in 2020. More than 244 million people were living with ischemic heart disease that year, more than 62 million had ischemic stroke, and almost 19 million had intracerebral hemorrhage.
Current guidelines recommend estimating 10-year risk of major adverse cardiovascular disease events to establish who should get a statin for primary prevention. This risk is calculated using the atherosclerotic cardiovascular disease (ASCVD) risk score, a statistical model that considers a host of variables, including age, sex, race, systolic blood pressure, hypertension treatment, smoking, Type 2 diabetes and blood tests. Statin medication is recommended for patients with a 10-year risk of 7.5% or higher.
“The variables necessary to calculate ASCVD risk are often not available, which makes approaches for population-based screening desirable,” Weiss said. “As chest X-rays are commonly available, our approach may help identify individuals at high risk.”
He added that, “We’ve long recognized that X-rays capture information beyond traditional diagnostic findings, but we haven’t used this data because we haven’t had robust, reliable methods. Advances in AI are making it possible now.”
A team at University of Utah, for example, earlier this year described how they used AI to predict the onset and course of cardiovascular disease in collaboration with Intermountain Primary Children’s Hospital.
Compelling evidence
In the current study, Weiss and his colleagues developed the CXR-CVD model to predict the risk of death from cardiovascular disease using 147,497 chest X-rays from 40,643 participants in the Prostate, Lung, Colorectal, and Ovarian Cancer Screening Trial, a multi-center, randomized controlled trial designed and sponsored by the National Cancer Institute.
The researchers also tested the model using a second independent cohort of 11,430 outpatients (mean age 60.1 years; 42.9% male) who had a routine outpatient chest X-ray at Mass General Brigham and were potentially eligible for statin therapy.
Of 11,430 patients, 1,096, or 9.6%, suffered a major adverse cardiac event over the median follow-up of 10.3 years. There was a significant association between the risk predicted by the CXR-CVD model and major cardiac events.
The researchers also compared the prognostic value of the model to the established clinical standard for deciding statin eligibility. This could be calculated in only 2,401 patients (21%) due to missing data (e.g., blood pressure, cholesterol) in the electronic record. But for this subset of patients, the CXR-CVD risk model performed similarly to the established clinical standard and even provided incremental value.
“The beauty of this approach is you only need an X-ray, which is acquired millions of times a day across the world,” Weiss said. “Based on a single existing chest X-ray image, our deep learning model predicts future major adverse cardiovascular events with similar performance and incremental value to the established clinical standard.”