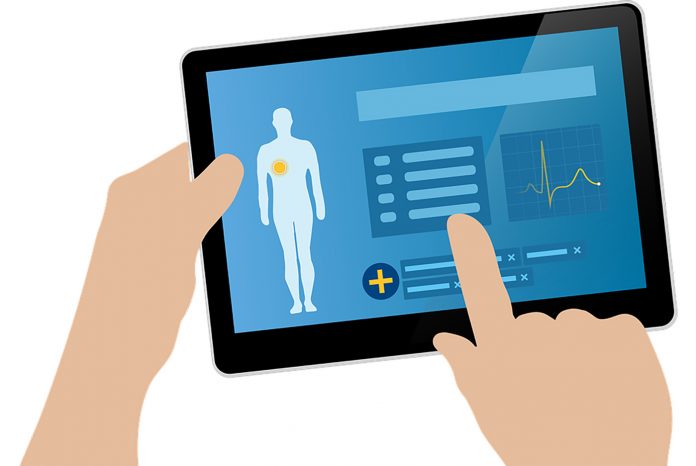
Artificial intelligence can filter through electronic health records (EHRs) to identify patients with rare, undiagnosed diseases, research suggests.
A machine-learning algorithm was able to spot more than half of individuals who went on to be diagnosed with the inherited disease of common variable immunodeficiencies (CVID).
The group of immune disorders leaves patients susceptible to infections and autoimmunity and is characterized by antibody deficiency and impaired B cell responses.
The findings are published in the journal Science Translational Medicine.
“Immune deficiency patients elude diagnosis for 5–10 years because of the heterogeneity of clinical presentation, and our AI enables early diagnosis,” researcher Manish Butte, from the University of California at Los Angeles (UCLA), told Inside Precision Medicine.
“The impact will be to reduce morbidity and mortality, reduce costs, and reduce unnecessary testing.”
At least 68 genes are implicated in CVID, one of the most common inborn errors of immunity (IEI), but for most individuals no specific genetic cause can be identified.
Because of the variety of clinical IEI presentations, patients with CVID can initially present to a wide range of clinical specialists who focus on the specific organ system involved, such as the lung or liver, rather than directly to an immunologist for the underlying immune defect.
This organ-based approach can result in tunnel vision and hinder a formal diagnosis in IEI, the authors note, particularly for those patients who have multisystem manifestations that fluctuate over time and it may result in diagnostic delays of a decade or more.
In an attempt to improve on this situation, the researchers used a machine learning approach to identify phenotypic patterns of the CVID phenotype, or EHR signatures, encoded in patients’ medical records.
They then trained an algorithm to identify patients who likely have CVID but who have been “hiding” in the medical system.
As CVID does not have a single clinical presentation, constructing an EHR signature for the disease phenotype was not straightforward, the investigators note.
They developed a computational algorithm called PheNet that gleans EHR signatures from the records of patients with CVID and computes a numerical score that prioritizes patients most likely to have the condition.
To test whether they could shorten the diagnostic odyssey of CVID, Butte and co-workers examined patients’ PheNet scores, looking back in time in the EHR data.
They examined the ability of PheNet to find previously undiagnosed patients with CVID in a large UCLA dataset of approximately 880,000 individuals, with data divided into 80% training and 20% testing, and performed blinded chart reviews of the top-ranked individuals.
They found that PheNet could have diagnosed 64% of the 58 patients with at least one year of EHR data before their diagnosis under the International Classification of Diseases.
PheNet could identify these individuals as likely having CVID a median of 244 days before their ICD diagnosis.
To demonstrate its ability to learn locally and act broadly, the team applied PheNet to more than 6 million records across five disparate health systems in California and Tennessee.
They found that in the top 100 ranked individuals on PheNet, the average enrichment for CVID diagnosis across all six institutions studied was around 434 fold over what would occur with random ranking.
“Artificial intelligence and machine learning are rapidly entering into the medical realm,” the researchers note.
“We show that approaches such as PheNet can learn from the EHR both to expedite the diagnosis of patients with CVID and to identify phenotypic patterns of rare diseases.”