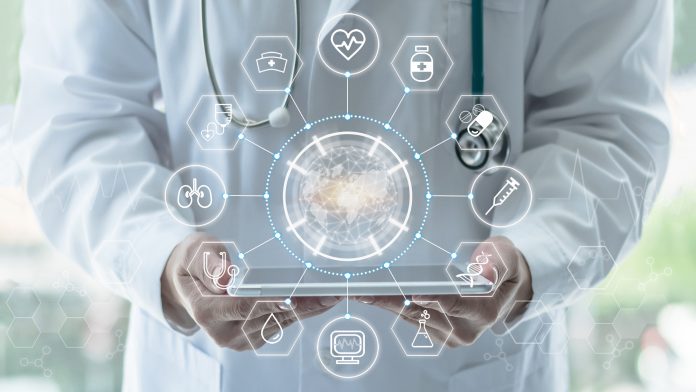
An adaptive artificial intelligence (AI) technology called the Targeted Real-time Early Warning System (TREWS) developed by Bayesian Health has shown its ability in a clinical setting to be an effective early warning screening tool for patients with sepsis. Detailed in three separate studies published in Nature and npj Digital Medicine, researchers from Bayesian and Johns Hopkins University demonstrated that deployment of the AI-driven clinical sepsis screening approach reduced mortality, morbidity, and length of stay of hospital patients. Importantly, the studies show timely use of Bayesian’s AI platform is associated with a relative reduction in mortality of 18.2% in sepsis patients.
Sepsis is the leading cause of death in hospitals across the United States, with one study estimating it accounts for—or plays a role in—as many as 35% of all in-hospital deaths. While this is a well-known problem, solutions for how to reduce sepsis-related mortality have been elusive due to the relatively few treatment options available, and also a lack of consensus among providers on the best treatment methods.
Early diagnosis key to improved care
“There is agreement that early recognition and treatment are critical to successful outcomes. Early administration of broad-spectrum intravenous antibiotics, in particular, is associated with decreased mortality and morbidity,” the researchers wrote in their study, “Prospective, multi-site study of patient outcomes after implementation of the TREWS machine learning-based early warning system for sepsis,” published in Nature Medicine. “However, heterogeneity in the presentation of sepsis often makes early recognition challenging, and many patients receive delayed care. This has spurred interest in the development of automated sepsis early warning systems to help clinicians recognize sepsis as early as possible.
For the current study, conducted with Johns Hopkins, the investigators used data from 764,707 patient encounters—17,538 of the patients with sepsis—across five hospitals in both academic and community-based hospital settings to test the AI-driven clinical sepsis screening approach. With more than 2,000 physicians using the TREWS software, the study achieved accurate early detection (1 in 3 cases were physician confirmed) at high sensitivity (82%) and significant lead time (5.7 hours earlier), high provider adoption (89%) with associated significant reductions in mortality, morbidity, and length of stay.
“There aren’t many things left in medicine that have a 30% mortality rate like sepsis,” said Neri Cohen, MD, PhD, president of the Center for Healthcare Innovation, a collaborator with Bayesian. “What makes it so vexing, is that it is relatively common and we still have made very little progress in recognizing it early enough to materially reduce the morbidity and mortality. To reduce mortality by nearly 20% is remarkable and translates to many lives saved.”
Providers “partner” with technology
The high provider adoption was especially notable, as one of the hurdles to the deployment of AI-based tools in the clinical setting has been how widely they are used once deployed. In the study published in npj Digital Medicine, the researchers noted: “While a growing number of machine learning (ML) systems have been deployed in clinical settings with the promise of improving patient care, many have struggled to gain adoption and realize this promise. Based on a qualitative analysis of coded interviews with clinicians who use an ML-based system for sepsis, we found that, rather than viewing the system as a surrogate for their clinical judgment, clinicians perceived themselves as partnering with the technology.”
“While we all understand the value of leveraging AI to improve the delivery of care, achieving measurable impact has proven to be much harder than advertised,” said Suchi Saria, PhD, CEO of Bayesian Health and director of machine learning, AI, and healthcare lab at Johns Hopkins University. “These results showing high physician adoption and associated mortality and morbidity reductions are a milestone for the field of AI and are the culmination of nearly a decade of significant technological investment, deep collaboration, the development of novel techniques, and rigorous evaluation.”
Bayesian’s adaptive AI technology integrates with a hospital’s electronic medical record (EMR) where it can provide actionable early detection flags and other key patient insights. These warning flags are shown on the patient list and can be linked with paging, phone, or other escalation pathways to alert clinicians. When Bayesian’s adaptive AI suspects a patient is at risk of developing sepsis, it immediately alerts doctors and nurses through the patient’s EMR, and then prompts the provider to take specific actions, such as requesting blood cultures or prescribing antibiotics.
“Bayesian’s AI-based technology overcomes common hurdles faced by many physicians by using cutting-edge strategies to increase precision, strengthen models, and encourage behavior change and ongoing use,” Cohen noted. “As a result, it provides technology accuracy that is 10x higher than other solutions in the marketplace.”